Migraine is one of the world’s most debilitating disorders, and recent studies have shown that changes in the retina can be a potential biomarker for the disease. Advanced imaging techniques like Optical Coherence Tomography (OCT) and Optical Coherence Tomography Angiography (OCTA) can help in measuring retinal thickness and vessel density, providing insights into microvascular changes that might serve as biomarkers for migraine. Notably, studies have found that migraine sufferers often exhibit reduced retinal thickness and lower vessel density, with more pronounced changes observed in those with chronic migraine or migraine with aura.
The Curtin Institute for Data Science (formerly known as the Curtin Institute for Computation) was brought in to address a significant technical challenge faced by researcher Virginie Lam: extracting and analysing data from a large set of historical OCTA scans, which were only available as PNG screenshots. The existing MATLAB code was inadequate, as it did not capture all necessary parameters and contained several errors. The task at hand was formidable, involving approximately 2560 images from an 80-patient cohort, which previously required manual processing by human researchers.
Our team developed a Python-based Optical Character Recognition (OCR) pipeline to automate the data extraction process from these images. The OCR technology was crucial for converting text and numerical data embedded in the images into a digital format, suitable for further analysis.
In addition to automating the extraction, the project explored the possibility of direct data extraction methods that might bypass OCR, aiming for even greater efficiency and accuracy. After the data was extracted, it was rigorously quality-checked and compiled into an Excel format, making it easier for further analysis.
The project was highly successful, with the Curtin Institute for Data Science team delivering accurate and reliable data extraction from the existing image backlog. This achievement not only advanced research into the retinal vascular changes associated with migraines but also demonstrated the potential for applying similar methodologies to other areas of medical imaging. The project lead expressed great satisfaction with the outcomes, noting that the project significantly enhanced data accuracy and processing efficiency. This project highlights the value of interdisciplinary collaboration between data science and medical research.
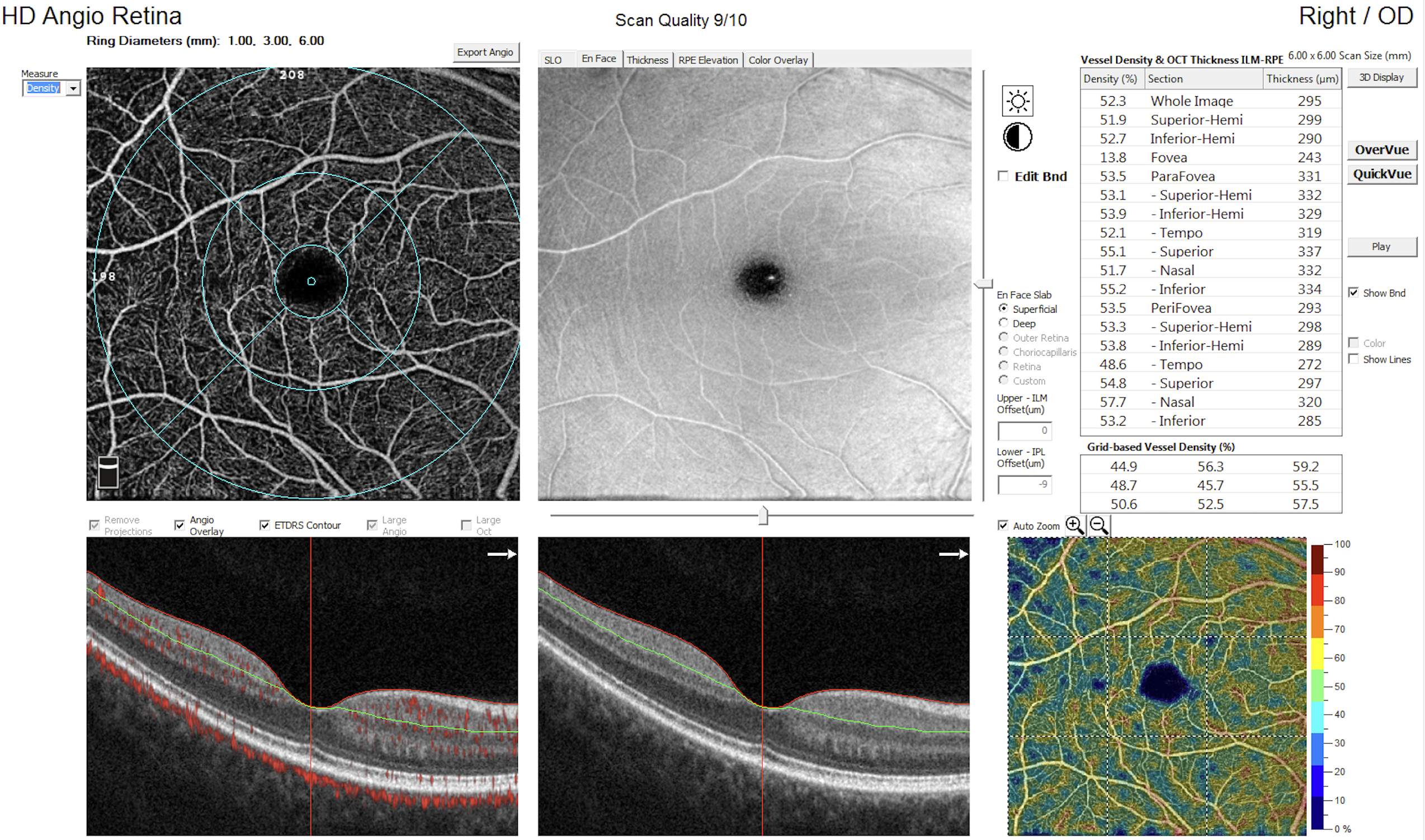